Overview
This dataset contains high-resolution aerial imagery from the USDA NAIP program [1], high-resolution land cover labels from the Chesapeake Conservancy [2], low-resolution land cover labels from the USGS NLCD 2011 dataset [3], low-resolution multi-spectral imagery from Landsat 8 [4], and high-resolution building footprint masks from Microsoft Bing [5], formatted to accelerate machine learning research into land cover mapping. The Chesapeake Conservancy spent over 10 months and $1.3 million creating a consistent six-class land cover dataset covering the Chesapeake Bay watershed. While the purpose of the mapping effort by the Chesapeake Conservancy was to create land cover data to be used in conservation efforts, the same data can be used to train machine learning models that can be applied over even wider areas. The organization of this dataset (detailed below) will allow users to easily test questions related to this problem of geographic generalization, i.e. how to train machine learning models that can be applied over even wider areas. For example, this dataset can be used to directly estimate how well a model trained on data from Maryland can generalize over the remainder of the Chesapeake Bay. Python code for training and testing deep learning models (Keras/TensorFlow based) can be found in the accompanying GitHub repository:https://github.com/calebrob6/land-cover
Further developments in models and related tools can be found at:https://github.com/Microsoft/landcover
Papers using a superset of this data include [6, 7]. Paper [8] uses data from the same sources.Citation
If you use this data set, please cite the associated manuscript:Robinson C, Hou L, Malkin K, Soobitsky R, Czawlytko J, Dilkina B, Jojic N. Large Scale High-Resolution Land Cover Mapping with Multi-Resolution Data. Proceedings of the 2019 Conference on Computer Vision and Pattern Recognition (CVPR 2019). (bibtex)
Dataset organization
Tiles
This dataset is organized into non-overlapping tiles. A tile is a spatial area measuring roughly 6km x 7.5km (with definitions that roughly match up with USGS quarter quadrangles). Each tile comes with seven corresponding GeoTIFFs:- NAIP 2013/2014 imagery (“_naip-new.tif” suffix)
- NAIP 2011/2012 imagery (“_naip-old.tif” suffix)
- Chesapeake Conservancy land cover labels (“_lc.tif” suffix)
- NLCD 2011 labels (“_nlcd.tif” suffix)
- Landsat 8 leaf-on composite (“_landsat-leaf-on.tif” suffix)
- Landsat 8 leaf-off composite (“_landsat-leaf-off.tif” suffix)
- Building footprint mask (“_buildings.tif” suffix)
- Delaware 2013 (only 107 tiles)
- New York 2013
- Maryland 2013
- Pennsylvania 2013
- West Virginia 2014
- Virginia 2014
- wv_1m_2014_extended-debuffered-train_tiles/
- wv_1m_2014_extended-debuffered-val_tiles/
- wv_1m_2014_extended-debuffered-test_tiles/
Data description
The contents of each type of data layer are as follows:- NAIP – Four-channel rasters that contain the R, G, B, and NIR bands respectively. Values are uint8s.
- Landsat 8 – Nine-channel rasters that contain the B1, B2, B3, B4, B5, B6, B7, B10, and B11 bands from a median mosaic of Landsat 8 surface reflectance imagery. The bands are described here. Values are float32.
- Chesapeake Conservancy land cover labels – Single-channel rasters that contain high-resolution land cover labels from this dataset. The values are uint8s with the following mapping:
- 1 = water
- 2 = tree canopy / forest
- 3 = low vegetation / field
- 4 = barren land
- 5 = impervious (other)
- 6 = impervious (road)
- 15 = no data
- NLCD labels – Single-channel rasters with values that match those described here. The values of 0 and 255 indicate no data.
- Building footprint labels – Single-channel rasters with a binary mask generated from the Bing Building Footprints dataset.
Spatial index
This dataset includes a “spatial_index.geojson” file that contains the boundaries of each tile in the dataset in the EPSG:3857 projection with attributes indicating which split the tile is in as well as attributes that include the filename pointer for each layer of data (e.g. “naip-new”, “naip-old”, “nlcd”, …).Download links
A zipfile of the dataset is available at the following locations: cvpr_chesapeake_landcover.zip (GCP link, 140GB)cvpr_chesapeake_landcover.zip (AWS link, 140GB)
cvpr_chesapeake_landcover.zip (Azure link, 140GB) The MD5 checksum of the zip file is 0ea5e7cb861be3fb8a06fedaaaf91af9 . Data is already unzipped and available in the following cloud storage folders; see LILA’s direct image access guide for download instructions.
- gs://public-datasets-lila/lcmcvpr2019/cvpr_chesapeake_landcover (GCP)
- s3://us-west-2.opendata.source.coop/agentmorris/lila-wildlife/lcmcvpr2019/cvpr_chesapeake_landcover (AWS)
- https://lilawildlife.blob.core.windows.net/lila-wildlife/lcmcvpr2019/cvpr_chesapeake_landcover (Azure)
Contact
For questions about this dataset, contact calebrob6+lcmcvpr2019@gmail.com.Licensing
Labels The organizations responsible for generating and funding this dataset make no representations of any kind including, but not limited to the warranties of merchantability or fitness for a particular use, nor are any such warranties to be implied with respect to the data. Although every effort has been made to ensure the accuracy of information, errors may be reflected in data supplied. The user must be aware of data conditions and bear responsibility for the appropriate use of the information with respect to possible errors, original map scale, collection methodology, currency of data, and other conditions. Credit should always be given to the data source when this data is transferred, altered, or used for analysis. Images Landsat and NAIP imagery has been released into the public domain. License information about NAIP and Landsat is available here and here, respectively. Building footprints The building footprints are licensed under the Open Data Commons Open Database License (ODbL). License information about Microsoft’s US building footprints dataset isavailable here.References
- United States Department of Agriculture. National Aerial Imagery Program. Online.
- Chesapeake Conservancy. Land cover data project. Online.
- Homer C, Dewitz J, Yang L, Jin S, Danielson P, Xian G, Coulston J, Herold N, Wickham J, Megown K. Completion of the 2011 National Land Cover Database for the conterminous United States – representing a decade of land cover change information. Photogrammetric Engineering & Remote Sensing. May 2015.
- United States Geological Survey. Landsat 8. Online.
- Microsoft. US Building Footprints. Online.
- Malkin K, Robinson C, Hou L, Soobitsky R, Czawlytko J, Samaras D, Saltz J, Joppa L, Jojic N. Label super-resolution networks. International Conference on Learning Representations (ICLR). 2019.
- Robinson C, Hou L, Malkin K, Soobitsky R, Czawlytko J, Dilkina B, Jojic N. Large Scale High-Resolution Land Cover Mapping with Multi-Resolution Data. Computer Vision and Pattern Recognition (CVPR). 2019.
- Robinson C, Ortiz A, Malkin K, Elias B, Peng A, Morris D, Dilkina B, Jojic N. Human-Machine Collaboration for Fast Land Cover Mapping. arXiv 1096.04176, June 2019.
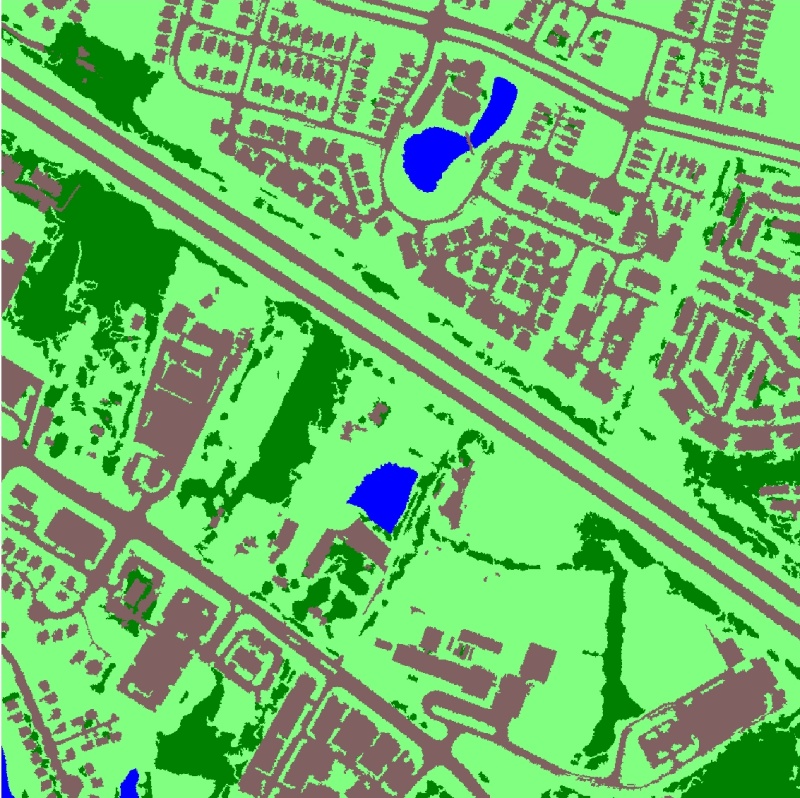